It’s easy to get swept into the hype of big data and AI with all the strides being made in industrial manufacturing and predictive maintenance.
Less easy is figuring out when, how, or even if your own business could integrate data-driven strategies to gain a competitive advantage. Or if the insights provide the kind of ROI that justifies making it happen.
We get approached all the time by organizations putting the cart before the horse — they’re thinking “AI is important, we should figure out how to use it.” The thinking should instead be “What is a business problem I am trying to solve? And is AI the correct way to solve it?”
We’re here with a guide to help you through that process, and will explain how an organization like Predii connects any missing dots.
First, the basics of machine learning
Mark Bishop, a professor of cognitive computing, says that machine learning applies to “any type of question that takes a few moments for a human, repetitively, and has clear associative links between some parameters in data.” When it comes to business, implementing machine learning is the process of using the past to make targeted predictions about the future. By analyzing a large set of historical outcomes we can look at new inputs and make causative predictions.
Generally, these results could be calculated by humans — the advantages of machine learning come from the exponential speed at which the results are produced.
Seems simple, right? And yet there are examples of organizations all over the world that are finding it incredibly hard to implement data-driven principles. Murli Buluswar, AIG’s Chief Science Officer, makes the point that the biggest hurdle is mindset, not resources.
“The biggest challenge of making the evolution from a knowing culture to a learning culture — from a culture that largely depends on heuristics in decision making to a culture that is much more objective and data driven and embraces the power of data and technology — is really not the cost. Initially, it largely ends up being imagination and inertia.”
What he’s saying is that implementing machine learning can be uncomfortable for organizations because it may initially appear to disenfranchise individual expertise — nobody wants to lose their niche. However, like he said, this becomes largely a question of imagination, as roles shift from descriptive and diagnostic to predictive analytics.
How do I integrate this with my own enterprise?
The first thing you need to do is ask two questions:
What are my KPIs?
What process related to them happens more than once?
Machine learning thrives with repetitive processes, second nature tasks that feel automatic where large amounts of people doing the same thing get largely the same results. The question is how to assess your KPIs to figure out which involve tasks that machine learning can optimize.
Segmenting the tasks involved in your job or enterprise is the first step. Separate tasks that require lots of thought from those that are automatic, tasks that are infrequent or unpredictable from tasks that happen frequently, and tasks with unpredictable outcomes from ones with predictable outcomes.
The tasks that are automatic, occur frequently, and have predictable outcomes are more viable for machine learning than others.
Now the question is whether you have been tracking this task. Do you have any data? If the answer is no, what processes can you implement to start collecting it immediately? Is the data relevant to your task? If it’s a repair problem you’re trying to solve, you need a sizeable database of repair records. If it’s a manufacturing efficiency problem, you need to fully track all the factors involved in your throughput. The context of your data is just as important as the quantity of it.
Once you have the data, you need to figure out how to use it.
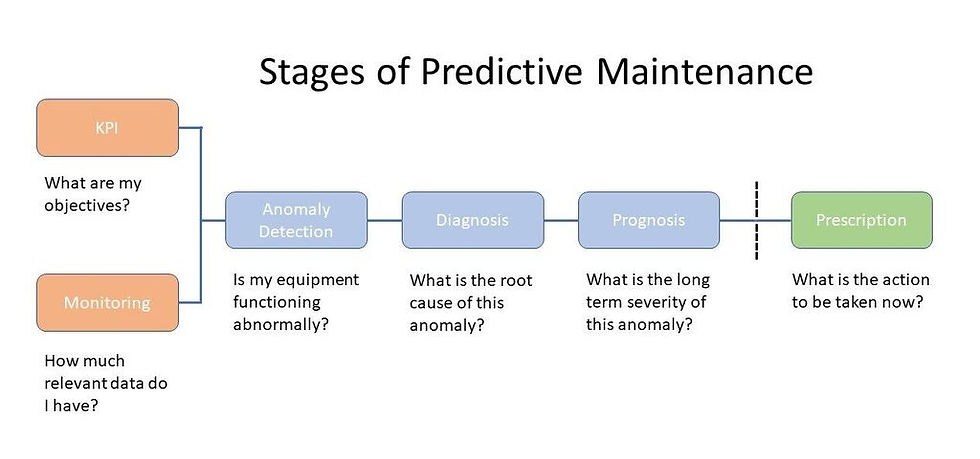
What do you do with your data?
Data in a vacuum helps nobody. Quoting statistics from NewVantage Partners, Charles McLellan confirms that “the main cultural impediments to big data adoption lie with business units rather than the IT department,” saying what we know already: having the data is great, but using it is the real challenge. To make a medical analogy, being diagnosed in and of itself is unhelpful. The diagnosis is only useful because it leads to the prescription that cures the illness.
Frequently, getting use of data means hiring new people to your team to process it. Let’s go back to Murli Buluswar and AIG. You can read about their experience implementing evidence based decision making here. Of particular note is the team they created to do it: “behavioral economists, psychologists, engineers, and change management experts work hand-in-hand with data scientists, mathematicians, and statisticians.”
Not every organization has the bandwidth to create that kind of team, and that’s where Predii comes in. Predii provides all the tools and industry knowledge required to take your enterprise from heuristic, intuition-based management to a data-driven competitive strategy based in objective information.
We find meaningful patterns in your data: we specialize in assimilating knowledge from large data sets that fall outside the bounds of normal human language or cognitive capacity and making this usable for the enterprise today. Our solution is called “Unnatural Language Processing™” — what does it mean when a customer says their car “creaks like a used La-Z-Boy?” Is it the same as another customer who says their car “squeaks like their grandmother’s knees?” Our platform connects the variables. This analysis leads to quick, actionable insights, allowing your business to eliminate guesswork, maximize uptime, detect anomalies, eliminate false positives, streamline operations, guide repairs, preemptively service equipment, accelerate diagnostics — just a few examples from our suite of solutions.
コメント